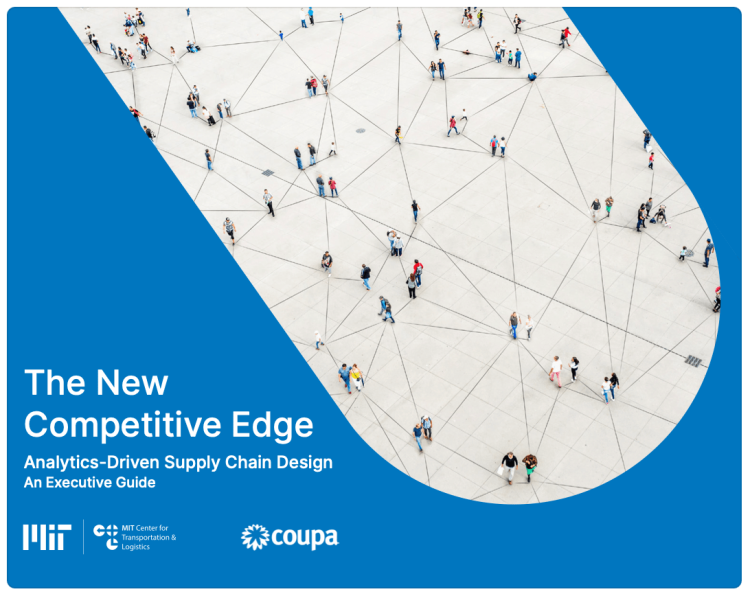
In supply chain design, also called strategic supply chain planning, senior management makes decisions regarding resource investments, business policies, and deployment issues in redesigning their supply chains. Not surprisingly, these decisions have a major impact on operational efficiency and competitiveness.
The supply chain design field is rooted in conventions developed through research carried out in the 1990s. These studies focused on the physical structure of supply chains (i.e., decisions related to the number, location, capacity, and mission of the company’s production-distribution facilities and flows between different parts of the network). Designs were typically reviewed every few years over a long planning horizon and were usually the responsibility of the supply chain or logistics department or entrusted to a dedicated supply chain analytics or modeling team.
But the commercial environment in which today’s supply chains compete is very different from the one that existed three decades ago—and it continues to evolve rapidly. Consequently, traditional design methods have become outdated, and companies that follow them risk putting themselves at a competitive disadvantage. However, there are tremendous opportunities for enterprises that take a more enlightened path. As markets have evolved rapidly over recent decades, so have supply chain design practices and tools. Companies can capture significant gains in operational efficiency and competitiveness by taking advantage of these advances and updating how they approach supply chain design.
The following sections show how these gains can be realized.
Current Approaches to Supply Chain Design
Supply chain design is supported by analytics approaches that can be embedded in decision-making tools and fed by relevant supply chain data. The following is a brief overview of the state-of-the art and state-of-practice in these areas.
Analytics Approaches
Supply chain design has typically been supported by two prescriptive analytics approaches: optimization approach and simulation approach. In practice, a combination of the two approaches can be beneficial.
The optimization approach typically employs mixed-integer linear programming (MILP) models to establish network configurations (e.g., number and location of facilities or structure of flows between different parts of the network) that aim to maximize some measure of performance while taking into account certain business constraints (e.g., limitations about the capacity of different facilities). These models can either be used to perform a “greenfield” optimization, where the objective is to determine an optimal design for a new network that does not incorporate existing infrastructure, or a “brownfield” optimization, where the focus is on adjusting the design of an existing supply chain network while keeping some of the existing design intact.
The simulation approach aims to assess the expected performance of a supply chain design based on various inputs, such as by establishing total cost to serve for a given network configuration. While the simulation approach (unlike the optimization approach) does not guarantee that the optimal network performance will be reached concerning a specified performance metric, it typically is significantly less expensive from a computational standpoint. This allows decision-makers to incorporate more refined input data and more complex relationships between different network elements.
Data and Decision-Making Tools
To harness the full potential of supply chain design, organizations can rely on huge amounts of data and employ decision-making tools of various degrees of sophistication. However, we find that collected data is not always used to support supply chain decision-making and that the decision-making tools employed vary widely across organizations. In the following paragraphs, we describe how the data and tools organizations use differ according to their level of decision-making maturity.
At the most basic level of decision-making maturity, relevant data is dispersed throughout the organization and basic tools (such as spreadsheet analysis) are employed. The decision-making process is typically siloed and limited to supply chain functions.
As the decision-making maturity grows, organizations recognize the need for end-to-end decisions and cross-functional collaboration. They invest in tools to gain organizational visibility and enable collaboration between different teams.
In highly mature decision-making environments, we witness a move toward a single data repository, a common source of truth embedded into more sophisticated and widely distributed planning tools. These serve as major enablers of collaboration between different teams. Supply chain design decisions are no longer confined to one part of the organization but instead emerge from a multi-stakeholder decision-making process.
Using Planning Optimization for End-to-End Supply Chain Decisions
The typical pharmaceutical supply chain is long and complex. It often includes multiple stages of production that are interdependent across many locations around the world. A slight change resulting from a decision in one area, which might improve operations locally, could trigger unexpected impacts elsewhere, leading to higher costs and lower efficiency in the supply chain as a whole.
For example, to avoid the cost of additional shifts, a packaging team might decide to smooth out their production plan by pre-building some volume at a time when demand is lower, which might be weeks or months ahead of when it will be needed. This means the assembly and filling teams must also adjust their plans to provide the input materials, which might increase their production costs. Moreover, because of a large minimum order quantity (MOQ) for the batch size, they will have to make much more packaging than usual and hold excess inventory—while bearing the added inventory holding cost.
Consider the example of one pharmaceutical manufacturer. The company used to manage production planning with spreadsheets and emails. Different stages of production had their own planners, who would submit their individual plans in the third week of each month. Then the supply chain planning team scrambled to negotiate with the production teams and piece the different plans together for a feasible plan in time to make a decision before the end of the month. Because this process was very manual and prone to error, it typically resulted in suboptimal plans.
To avoid such errors and improve planning, Coupa’s Supply Chain Design and Planning (SCDP) team worked with the company to set up a repeatable optimization planning solution that covers the end-to-end supply chain, including primary and secondary manufacturing facilities as well as the distribution network to the company’s markets. This solution considers the dependent demand among different stages and recommends feasible solutions. More importantly, it can find a holistically and mathematically optimized production plan that balances production costs, inventory holding
costs, and transportation costs. Each production team now only needs to provide the refreshed inputs, such as run rates and capacity, in an agreed format. This feeds an automated data-processing workflow that validates and cleans the input data, then triggers the optimization and visualizes the recommendation of the optimized plan. The time required to refresh the production plan has been shortened from weeks to days. Planners can also run multiple “what-if” scenarios concurrently. As a result, rather than being tied up with spreadsheets and emails, the supply chain planning team can spend most of their time understanding the optimization results and making crucial decisions