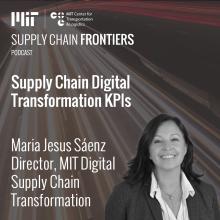
Today, on MIT Supply Chain Frontiers, editorial director, Ken Cottrill speaks with Maria Jesus Sáenz, director of the MIT Digital Supply Chain Transformation Lab, about how companies use artificial intelligence and machine learning to identify key performance and key learning indicators during digital transformations.
Sáenz shares examples of how companies may use AI and ML to collaborate on data models and leverage publicly available data to craft more accurate forecasts or discover hidden efficiencies. Learn more about how AI and machine learning are used in digital transformations at digitalsc.mit.edu.
Learn with Dr. Sáenz at MIT Supply Chain Bootcamp.
Transcript
- ♪ Up beat music ♪ [Narrator] Welcome to MIT supply chain frontiers from the MIT center for transportation and logistics. Each episode features center researchers and staff who welcome experts from the field for in-depth conversations about business, education and beyond. On today's episode CTL's Ken Cottrill speaks with MIT CTL research scientist Maria Jesus Saenz about how companies are using AI and machine learning to develop novel key performance and key learning indicators to train mathematical models during digital transformation. Take it away, Ken.
♪ Up beat music ♪ [Ken Cottrill] Uh, welcome to the podcast.
[Maria Jesus Saenz] Hello Ken, thank you very much for inviting me.
[Ken] In this episode, we're going to talk about artificial intelligence, um, machine learning in the context of key performance indicators and how that technology can improve supply chain KPI's. I mean, one of your presentations, you do say that, um, something like 75% of executives believe that functional KPI's should be achieved with AI and ML. So why is that?
[Maria] Yeah, this is a very interesting question. So I think that first we need to, to define what AI means in the context of supply chain management, right? So the idea is that a certain machine can be a device or could be a robot, or could be maybe an algorithm, performs cognitive functions that are typically associated with human minds. Like for example, perceiving or learning or interacting with environment or with the stakeholders. right. Problem solving. In that context, yes, for them machines to have anchor points in which they can interact in their decisions, becomes key because at the end, the machine needs some structure in order to propose whatever kind of position or whatever kind of analysis. So in that context, these anchor points are the KPIs. They keep it from us indicators. This is why in order to take advantage of all the effort that we made, uh, for all the investment that we've made behind of AI implementation or material learning implementation it's important to have a clear picture of what we expect. And here is where, I mean, KPIs has a very important role. Yeah?
[Ken] Right. Additionally, I would like to highlight that in a new digital era, we, uh in the perfect scenario of course we will have different layers of data, right? That will be connected, represented different units or entities could be, for example, an SKU how it's manufactured, how is from end-to-end deliver to my customer right? About this, this SKU has a logistics service provider, this helping in the last mile delivery on them uh, transportation one level. So in that regard, so it's so important that all of these layers of data are connected. And then we can put anchor point that are the KPIs in order to improve this process. So then first we need to connect these layers of data and then we need to put anchor points in order to improve the efficiency of all these processes that are connected. Why? [Ken] Okay. So this is why KPIs are so important for supply chain and also so important for, for AI and materials.
- Understand. So AI and ML can play a critical role in determining which KPIs are measured and, and how they are measured. So can you explain that and perhaps use wallet two examples, KPIs
- Are I have a key role in structuring the way AI and material learning fixed, right? So let's put an example in the forecast but this is a typical setting and supply chain where machine learning has been implemented. And then it's growing quite a lot. In that particular context the typical KPI will be what is my forecasting accuracy? Then we can allow the system we can allow the AI system just to emulate the human mind you know, to, to improve their predictions. Right? So then maybe we can start incorporating new information and new data sources that are coming from the exterior of the company, right? So let's say maybe they could come from a forecast tool, a weather forecasting. I mean, they could come from certain government policies. So in that regard, it's important that then we connect on these sources in order to be able to improve the forecasting accuracy. As then, for example, maybe we can measure what is the Delta, what is this improvement for passing accuracy after integrating, I mean outside sources of data, right? So then again, this is why I mean the combination of AI and machine learning assuming it's now my dynamic process that it should learn or has the opportunity to learn. Maybe others, they will not learn but there is the opportunity to learn from what from the performance itself, but also from incorporating new sources of data or maybe yes to interact with the insight of the human that could increase these cognitive capabilities that we just mentioned. Right? So then this is why, I mean the integration of AI and KPIs can have a very good wall working, working together. Maybe we can put another example, right? For example, let's say, imagine that we are creating a data platform in which we are promoting the collaboration of different retailers, call me from different segments. Let's say grocery with restaurant in the online delivery right? And example, dry cleaning services. That's not in the same geographic area. These three partners are different partners by nature with even with different KPIs in terms of their sales, because the natural of the product or the services are different. But in terms of the last mile delivery they have common KPIs. So can they share their logistics flows and can they share their KPIs in order to be more efficient in terms of the logistics can, for example they create a new KPI jazz designed to create density while they are corroborating, who is contributing in a higher manner to creating in this density, right? Maybe this partner could be incentivized if we integrate these new KPI based on density or the contribution to density. And then maybe we can connect these new KPI of density and contribution to density from each of the partners maybe even be increasing on or improving on on-time delivery. Right. That these they're real KPI of all these three partners working together in last mile delivery. Right? So these are like two examples about the beauty of KPIs and AI and machine learning but especially the different nature of KPIs in all these interactions. Right? So we can have KPIs that are more focused on the process itself, for example last mile delivery on time delivery, but also thinking about new set of KPIs that can be artificially created in order to trigger the improvement of the main main performance of that particular.
- Okay. So, so what you're saying is that in the platform example the AI ML technology would actually identify the opportunity for sharing density, for example. Yeah. And then, so by doing that then you would develop the KPI to measure the effectiveness of that feature. Yeah
- You are totally right. And maybe we can identify not only the KPI but also maybe the partner who is better contributing to improving this on-time delivery because maybe these parents creating more density or maybe this parent is providing more flexibility in the last mile delivery that could benefit on the partner benefit on the synergy of the three of them. So somehow we should recognize this contribution. Why can maybe to incentivize to in certain periods of time of peaks in the delivery, in this kind of platform maybe we could incentivize more flexibility, right. In order to reach more efficiency. So then we should mess up flexibility. This is the summary, right. Let's create a new KPI in terms of flexibility because it's helping in the field.
- So having to establish those KPIs using AI umbrella in that way, can the also be used to optimize the chaos you've already got you've you've actually you've actually established, can optimize those KPIs. Yes.
- Yes. For example, in this case imagine that we are having, let's say 100 partners can we focus on those partners, maybe clustering, right. Is an unsupervised material learning approach. Can the platform cluster in a dynamic manner, these partners for this weekend that maybe it's going to be busy due to the weather, and then can we cluster them in order to discover what are the best performance, right. And based on the best performance discovery what are the antecedents what are the other parameters that are triggering this kind of better contribution to a PCC? Right. So then, and then they really start optimizing just discovering patterns. Or maybe we can use another method, typically rise to be prescriptive finding the optimizing function. And then what is the, I mean formulation that is helping us just to optimize maybe including these new incentive for the flexibility. Right. So we are playing with it automate, I mean a performance KPI that maybe is on 10 delivery are what are in the middle or other KPIs, like that's easy contribution to density, contribution, to flexibility from the different partners that we could create a system of incentives in order to impact ultimately in in the own time. I don't.
- And you're also, I think by implication you're also saying that that that process will also or could also identify new KPIs that KPIs that the companies evolved never even used before. Yeah.
- So we have worked with some companies that are developing this kind of digital strategy focusing their attention on certain strategic vision. Right. I want to develop, for example a synchronized network, right? So, but how I measure this patient, how I measure how well is the degree of synchronization of my supply chain, right? So they can, I defined what synchronization means for me and for my suppliers. Can I transfer the new KPI to my suppliers in order to incentivize my joint synchronization with my suppliers then maybe measured in these kinds of monitors. And also what are the gaps? Maybe the gaps are with certain suppliers or maybe the gaps are worse in certain regions, or maybe answer certain disruptions. Let's try to focus on solving these gaps in synchronization in order to keep improving my, my Antwon supply chain right
- Now you mentioned digital strategy, where should this you know, the process of using AI and ML to improve KPIs? Where does that sit in a digital strategy? The
- This is a great question because what we have up circle. So with the company that we have been working with is that they think that these are kind of AI Matilda and he's a kind of cocktail Mateen which we are putting all our data. Let's allow the system the algorithm to discover because it's unsupervised, right? And the system will discover their way. This is not, the magic is not in that way. Right? So what we have observed that AI machine learning is so context dependent. So what we can apply for maybe certain problems categories can not be generalized to all the product categories, right. Because it depends for example how normal is my product in the market. Yeah. I mean, can be substitute with other products can be cannibalized by other problems or maybe the region is different in terms of forecasting in terms of demand because there is more uncertainty on lady their supply chain planners are not so good in training the system, right. So then is, this is the, the, the most difficult part because the effort and the investment you need to put it in order to bail out your machine learning AI system are huge. And then you are expected to a scale by generalization, but it's not the way you scaling is just by contextualization. Right? So then let's discover what are the context parameters in order to be able to translate to other contexts that they belong there that are patient to this context. And then maybe in some cases it needs just to read develop the material learning. So it's so context dependent. This is one one feature other features that is also very dynamic. So since we are expecting the material learning to land then we should assume that these are dynamic process that where we are integrating a forecasting system during the first weeks of the of the launching of the product is not the same that are that after 100 weeks, right? Or after one year, right? Or maybe if we are having another product that can be a mirror in terms of forecasting for this particular product. So again, it's context dependent porpoise dependent is dynamic. So we should be prepared for observing and capturing how the mature learning AI system evolves because learning should be the beauty right of the of the system. But also we should be prepared to embrace the opportunities of this learning by two, we should observe what is going on in this evolution of the AI.
- So as the algorithms learn then is it sometimes necessary to go back and revisit the KPIs and maybe modify, or even, even introduce new ones? Sometimes
- The system learns in a, in a biased way something that we don't expect in this particular case we need to especially at the beginning, right. We need to observe very carefully how the assistant evolves right. Just to make sure that the ball's in the right direction yes. To monitor what are the outcomes. Yes. The expert even to help the system to put the right parameters, right. In order to help the system maybe to, okay now we are going to have, we expect a certain disruption. So then because certain government in certain country is going on, make certain announcements so let's be prepared right. For this. And so then somehow we need to, to to guide the system, especially at the beginning. So yeah. We need to come back sometimes. And that, to assume that sometimes is is we could have mistakes in this prediction or in the behavior of this
- You don't get very good point that the environment is very dynamic. And we've certainly seen that in the past, you know year or two with COVID. But does that imply that AI ML will become even more important in improving KPIs?
- Yeah, I think so. Because then what we would expect is to have more and more data, right. And the way of structuring understanding the data and taking advantage of this data is putting, I mean, different sets of KPIs. Yeah. So then of course we will continue making decisions with a more traditional KPIs in the kind of leveler mode but maybe answer, sir, under certain circumstances quite maybe we could focus more on how we could solve a particular disruption. So let's put a zoom there and then let's display all, let's deploy all other, I mean KPIs that could generate certain scenario. Right. And then we will go up again and then let's continue the regular mode and then let's go deeper because we are going to launch a new product. Right. So let's go deeper into more deployed KPIs. Right. And then we'll, we'll continue on, on, on that. Yeah. Kind of thing. Okay.
- Now you've provided a lot of great advice, really to listeners on some of the pitfalls to watch out for when you're applying AI and ML in this way. Is there anything else you'd like to mention in terms of challenges that companies should be aware of? So
- I will recommend think about the KPIs in a new way. It's not the more traditional way. Yeah. So for example, how we can monitor the improvement of my AI material learning in order to help my digital transformation journey. So there, I need to focus not only the KPIs but also in the Delta of the KPIs, would we call, you know research key learning indicators, right. In which we are monitoring the Delta the improvement of these KPIs and what are the different factors that are triggering with driving these improvement. So then these will help us yes. To scale the efforts that we are doing in mature learning or AI systems in the right direction because we will discover how the system is evolving. So yes, again, these different layers of KPIs in terms of the process that are being supported by AI but also key ally skill learning indicators how my transformation is being progressing because also this monitoring, this improvement is going out. So increase the motivation or the people who is affected by digital transformation. And also we can transfer lessons learned to other contexts. This will be one advice. Or the important advice is that sometimes implementing AI machine learning is a new way of thinking. So in some cases we need to redesign where our supply chain processes because the way of thinking the way of making decisions could change the way your, for example implementing end to end supply chain planning. Maybe it will change the way of communicating with my commercial department in order to have a sales and operation planning. Since we are gonna have very rich flow of data will change. Yes. So then it's not only gastric translating what we are doing right now. We are with our current operations or our current processes is that we need to, I mean sometimes to redesign the process from scratch with a white piece of paper and saying, okay how we are expecting to run this process. We base opportunities driven by data by AI machine learning.
- Understand good advice. That was great. I really appreciate your time. Thank you. Thank
- You very much for inviting me. Pleasure.
- All right, everyone. Thank you for listening. I hope you enjoyed this edition of MIT supply chain frontiers. My name is Arthur growl communications officer for the center. I invite you to visit us anytime at ctl.mit.edu or search for MIT supply chain frontiers on your favorite listening platform. Until next time.