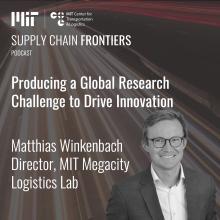
The MIT Center for Transportation & Logistics (MIT CTL) and Amazon engaged with a global community of researchers across a range of disciplines, from computer science to business operations, to supply chain management, challenging them to build data-driven route optimization models leveraging massive historical route execution data and machine learning models.
While we congratulated the winning teams and all participants in the news, Dr. Matthias Winkenbach joins today's Frontiers to share some insights and outcomes that the production of a research challenge brought about.
He speaks about how convening researchers from across all levels of academia and supplying rich data and a compelling problem, may drive more new research in areas of inquiry that are sparsely published on.
Learn more about the Challenge: https://routingchallenge.mit.edu/
Learn more about the Megacity Logistics Lab: https://megacitylab.mit.edu/
Transcript
Announcer:
Welcome to MIT Supply Chain Frontiers from the MIT Center for Transportation and Logistics. Each episode features center researchers and staff who welcome experts from the field for in-depth conversations about business education and beyond. Today, Ken Cottrill speaks with MIT CTL research scientist Matthias Winkenbach about the recently completed Amazon Last Mile Routing Research Challenge, where teams form from around the world to compete and build data-driven route optimization models, leveraging massive historical route execution data. Take it away Ken.
Ken Cottrill:
Welcome everyone to another Frontiers podcast. Today our guest is Matthias Winkenbach, an MIT CTL research scientist and director of the MIT Megacity Logistics Lab. So welcome Mathias.
Matthias Winkenbach:
Thanks, Ken for having me.
Ken Cottrill:
On July the 30th, you have announced the winners of the Amazon Last Mile Routing Research Challenge. Perhaps you can start us off today by describing the challenge, the rationale behind this, and how MIT CTL supported the project.
Matthias Winkenbach:
The Last Mile Routing Research Challenge was basically something that we have always wanted to do for many years already, because we're coming from a traditional operations research-driven field, right? Where route planning is predominantly an optimization exercise, but throughout the years with various projects, with various industry partners, we realized that optimization gets you far, but it doesn't get you as far as you would want to because of the true complexities that you are encountering in a real-world operational environment. There are just so many things that are very hard if not impossible to encode in an optimization model. And that's where the idea came up to think of data and to think of machine learning as a set of tools to unlock those additional 20, 30, 40% of quality in route plans that you could never achieve given your traditional toolbox of operations research methods.
But in a way we never really found the right partner to do it until we realized that Amazon actually had a similar research interest in us and was also basically curious to learn more from their own data about what the good route actually looks like and how the data that they have about their routes and about the deliveries that they're making on a daily basis could be used to further improve the quality of their routes.
And by improving the quality of a route when not necessarily speaking about coming up with cheaper or faster routes, because that's what traditional methods very much focus on, right? You have the single objective, which is usually minimizing costs, or minimizing distance, which never really kept just the full picture, never really captures everything that you want to achieve in a good route. Because a good route plan is also one that is, for instance, perceived well by the driver. That allows the driver, for instance, to find suitable parking. That allows the driver to operate safely. And last but not least also allows the driver to ideally avoid traffic in the sense that you don't go into a highly congested area if you already know that during that time of the day, it's going to be very hard to maneuver that space. And all of this, as I said, it's very hard to encode in traditional optimization methods. And that's why we wanted to go the machine learning route and try to come up with completely new ways or at least unconventional ways to think about route planning problems.
Ken Cottrill:
And part of that unconventional approach then is to use this sort of contest type approach where you will collect ideas from different teams, right?
Matthias Winkenbach:
Yes. I mean, in a way this challenge by itself is also a little bit unconventional because traditionally we would do research all by ourselves. So we would have an MIT research team working together with typically a corporate sponsor. And our team would basically do the research, work with the data of the sponsor, and ideally come up with our solution to the problem. And then this time, since this field is so new there's very little research being done, or at least being publicly available in this space of using machine learning in the context of route planning and route optimization. So we felt we, all by ourselves, will probably not come up necessarily with the best solution out there or the best ideas out there to basically kickstart this area of research which we believe has a lot of potentials. We came up with the idea of doing this as a challenge.
So basically to engage pretty much the entire research community to make it open to pretty much any researcher, any student out there, regardless of where they are, in which stage of their academic career they are. As long as they were academics and they were not doing this for commercial purposes, they were able to participate in this, get access to the same massive dataset that we would otherwise have access to exclusively, and basically try to compete for finding the best solution or try to compete and come up with the best possible ideas of how to tackle this particular challenge.
And I think this is an interesting approach to research because it crowdsources ideas. Our intention behind supporting Amazon with hosting this challenge was also not to let's say, find the ultimate solution to the route planning problem that we're trying to solve in this challenge, but it's rather about sparking ideas and also letting different members of a team or even different teams cross-pollinate each other with ideas on how to tackle the problem. And we're going to publish some of these ideas in later stages of the challenge as well so that we can basically bring it out to the world and let other researchers in the future build on these ideas to contribute to a growing research stream that hopefully eventually will get as much closer to what an actually good and sustainable and safe and efficient route planning approach would look like.
Ken Cottrill:
Now you mentioned machine learning, and I know that sort of distinguishes this project in many ways. Maybe you could just go into a little bit more detail as to how and why you use machine learning? What it actually brings to the table?
Matthias Winkenbach:
We obviously didn't quite know ahead of time how much it would actually be able to bring to the table. But the general idea behind encouraging people to use machine learning approaches to tackle this challenge was that we're living in a time where data about route operations throughout execution and also some of the environmental context of route execution is abundantly available. So it's not like we wouldn't know how drivers are operating their routes on a daily basis today. And we also have ways of accessing public data sources that tell us something about real-time traffic conditions or real-time weather conditions, things like that. But as I said before, traditional optimization-based methods have a hard time to systematically incorporate that information. And so to give you an example, if you look at route execution data for a certain area of demand across a larger time period, you will probably see patterns in there that are hard to explain based on pure optimization-driven thinking.
You will probably see that the drivers that operate in that area maybe avoid certain areas during certain times of the day even though that seems suboptimal, even though that seems as if it's adding additional mileage to their routes. But they're probably doing this for a reason and machine learning could actually help us detect those patterns and basically detect what drivers already know by looking at how they operate in this area.
So for instance, if drivers know that they can't find parking in a certain area during a certain time of the day, they would probably avoid this area during the time today anyway. And we can capture that information and incorporate that into our future route plans, such that the route plan doesn't even tell the driver to go there during that time of the day in the first place, but already accounts for the fact that for whatever reason, the driver doesn't like the area to make that time of the day. And similar logic could apply to other influences on safe and inefficient route operations like weather, like traffic, and other things, or even customer availability. Usually, the people on the ground know so much more about the individual customer, than your route planning IT system. And that kind of knowledge we would like to use to further improve future route execution.
Ken Cottrill:
So, okay. You've completed the project. So looking at this type of research generally, what do you think are the sort of pros and cons of this research method?
Matthias Winkenbach:
Well, one thing that we see for sure is that we are still at the very beginning of, let's say the use of data-driven methods and machine learning methods in what is traditionally known as hardcore optimization-based problems. So in many of the submissions, for instance, that we received, we saw that people are still trying to combine them, let's call them the old school operations research approaches with the new and upcoming machine learning-based approach. And that's probably fine. We probably want to combine the best of both worlds, right? Certain aspects of a route planning problem can probably still be most efficiently be tackled with a classical optimization approach. And maybe you just want to use machine learning to better calibrate these optimization approaches or to further improve an initial solution that you found using traditional optimization so, that's one thing that we see that we didn't really observe that many pure-play machine-learning solutions. We actually saw that many of the well-performing teams combined both types of methods.
We expect that a lot of research is going to come out of this in the next coming years because we're also going to make the data publicly available. So in the future, people can actually keep doing their research on this or related problems and benchmark it with the solutions that were found during this challenge. And hopefully, over time, people find better and better solutions and better and better methods to tackle these problems. And then the other aspect that is new to the way we ran this project was this crowdsourced approach, so this competition-based approach.
And here, I would say it's been very successful because at MIT we have the right resources in place to pull this off because in a way this was new for us as well. And luckily we had a great team, not just on the research side, but also on the pure technical backend side that was able to support a global challenge of this magnitude. And not every research group, not every university has the people and the resources to do that. And it's probably not, it's not required. We went for this approach because we wanted to kickstart this area of research and hopefully also other institutions around the world will benefit from this in the future.
But it comes with a high overhead of administrative effort and also the infrastructure that needs to be in place to actually run such a global challenge smoothly, reliably, and also fairly. So I'd say conducting research in this setup of a challenge will probably remain an exception. I don't see this happening on a daily basis in the future. But sometimes it could be the best way to spark the seeds for an innovative approach to a well-established problem. And now that we've done it once, I could see us do this on a somewhat regular basis. Maybe not every other month, but maybe every couple of years we might want to run such a competition again. Maybe on slightly different problems, maybe focusing on slightly different methods. But now that we have the infrastructure and the know-how in place, then I think we would be very well positioned to do this again.
Ken Cottrill:
So the depth of expertise, that's the lesson you learned? That obviously you need resources to be able to support this kind of project. Any other lessons that you learned that may be of use to any institution that might want to attempt a similar approach?
Matthias Winkenbach:
I mean, one important thing to keep in mind is obviously as I, as I said before, you want to make sure that you are able to cater to the needs of a potentially very large group of participants in such a challenge. Initially, when we started the signup period for this challenge, we had thousands of applicants, thousands of people who were eligible to participate in this. And obviously, throughout the course of such a challenge, you always have a massive dropout rate. So at the end of the day, we ended with a couple of hundred active participants out of this big pool of initial applicants, but still, this means you have to have the right resources in place to be able to respond quickly to any problem that may arise, but also to any kind of question that may arise. Because however hard you think about the problem ahead of time, there will always be questions that participants come up with that you haven't thought of before.
And you can only cater to a successful challenge if you're able to respond to such questions and inquiries quickly and efficiently. If you're not able to provide such a level of service, people would probably get dissatisfied with the challenge quite quickly and that might then put the entire success of the challenge at risk. And secondly, you want to be fair, right? You want to be able to communicate clearly what the objective of the challenge is. Aside from the pure research objectives, what are the people going to be evaluated on? And we actually spent quite some thinking around that. We first wanted to also give people a bonus if their solution was particularly innovative for instance. But then very quickly you run into the problem, how do you objectively evaluate innovation? Innovation could mean coming up with a completely new method, but innovation could also mean just smartly combining existing methods.
So which of the two would you prefer? And since we couldn't really answer that question for ourselves, we decided to go with the most objective evaluation criteria possible, which is a purely quantitative score that at the end of the day measures the quality of the route sequence that people came up with for basically a relatively large number of routes that they have to solve for.
And while this probably doesn't capture every single aspect of what might constitute a good or a not-so-good solution, it was the only way that we could think of to really do this evaluation objectively. And I think that's what counts if you're setting this up as a challenge, because otherwise if people feel that they're not evaluated fairly, that could be detrimental to the success of the challenge, but also to the perception of the challenge. So that's something to keep in mind if people want to set up competitions like this in the future. First of all, do you have the bandwidth to support this over a prolonged period of time for a potentially very large group of people? And secondly, how do you ensure that the way you evaluate the submissions is as fair as it could possibly be?
Ken Cottrill:
Far more complex than just a simple competition. And it sounds as if you learned a huge amount about the way you actually apply this kind of research. So what are the topics do you think will be really good candidates for this kind of approach?
Matthias Winkenbach:
That's a good question. I'm obviously coming from a supply chain point of view, and most importantly, I run a research lab at MIT that focuses on Last Mile Logistics. So most of the examples that I could think of are somewhat related to Last Mile delivery. This time we focused on routing. We could just as well think of maybe complex network design problems that could give rise to these things. But also if you think of inventory planning, which sounds boring because it's a very old discipline. But actually, now that we're seeing e-commerce going through the roof and people are getting used to things like same-day delivery, more and more. Suddenly inventory planning and inventory optimization become incredibly complex.
So this could be another one where I would say this could be an interesting challenge for future competition. But whatever it is, I think any complex planning problem that is heavily data-driven and computationally expensive so that you can't just solve simply with a simple linear program, for instance, would be a suitable candidate for this type of methodological approach, but also for a potential challenge-based approach to finding new methods of solving this problem.
Ken Cottrill:
Okay, so what about within MIT CTL? You'll work at the lab, you support a lot of different projects. Are there any projects that you're conducting now or would like to pursue within CTL that you think could be supported by this kind of approach?
Matthias Winkenbach:
I think a competition-based approach is most suitable for problems where you don't find a very vast body of academic literature yet. This is why we chose this route planning approach as our first subject for such a competition. Not because there is no literature on route planning or route optimization, actually there's been research on route optimization for decades. But the specific aspect of incorporating behavioral aspects, so basically trying to use data to understand human behavior better, and then incorporate that understanding into your planning problem. That's what sparked our interest in this in the first place. And that's what also gave rise to us setting this up as a competition. And if you translate this into other fields, for instance, a very hot topic these days is sustainability. And sustainability in logistics can be viewed from a lot of different angles.
So there's obviously an operational angle to it. So for instance, how do you plan supply chains or distribution networks to be set up for more sustainable distribution? Or how do you route vehicles in a more sustainable, more eco-friendly way? But actually, there's yet another behavioral aspect of this, which is consumer behavior. How do we incentivize consumers to behave in a certain way that allows us to serve them in a more sustainable fashion? And that is again something where there's plenty of data out there, but there are limitations to the way that we currently are able to model human behavior. And that might be something where again, a machine learning-based approach, for instance, could be an interesting methodological approach. And I believe hasn't been explored that extensively yet, but also where a competition-based research approach could be fruitful because there's simply not much out there yet.
I'm sure there's plenty of people around the globe who have started to think about this type of problem, but who probably haven't gotten far enough yet to really publish anything about this work. And very often the reason for people not yet published about it is that they're still lacking a suitable data set with which they can actually test their ideas.
And that's one of the big advantages of a competition that we ran this time. That we don't only put the problem out there, we also put a big and suitable data set out there that people can use to test their ideas. And that's what I mentioned earlier, right? This dataset will hopefully live on for many years as a benchmark set that people can use over and over again to test different ideas and to figure out which one works better and which one works worse. And I think similar approaches could be used for other supply chain and logistics problems that are in one way or the other affected by human behavior, human choices, and where we have readily available data that could be supplied as long as we find a corporate sponsor that is willing to supply that data.
Ken Cottrill:
You mentioned that the data is going to be publicly available. When do you anticipate that might be available?
Matthias Winkenbach:
So we're currently working on a literature review paper that's going to be published in the European Journal of Operational Research. And as part of that review paper we intend to actually make this dataset publicly available. So obviously this is a peer-reviewed outlet, so the exact timeline is very hard to predict. But we actually hope to have something out there that people can access and work with moving forward, but definitely before the end of the year.
Ken Cottrill:
So that will be a really interesting resource and a much sought-after resource as well. So Matthias, thank you very much for your time. Much appreciated and thanks for explaining this fascinating approach to research. I appreciate it.
Matthias Winkenbach:
Sure. Anytime. Thank you.
Announcer:
All right, everyone. Thank you for listening. I hope you enjoyed this edition of MIT Supply Chain Frontiers. My name is Arthur Grau, communications officer for the center. I invite you to visit us anytime at ctl.mit.edu or search for MIT Supply Chain Frontiers on your favorite listening platform. Until next time.